
The landscape of manufacturing is evolving at an unprecedented pace, marked by advancements in technology, automation, and the integration of smart systems. In this dynamic environment, the efficient coordination of tasks in job-shop manufacturing is crucial for maintaining operational excellence. Cranfield University offers an exciting opportunity for a self-funded PhD programme that focuses on Optimising Dynamic Scheduling in Smart Manufacturing through Reinforcement Learning.
About the Project:
The PhD project aims to revolutionise dynamic scheduling in job-shop manufacturing by introducing an adaptive self-organising solution. Led by Cranfield University, the research will leverage deep reinforcement learning and multi-agent systems to create a cutting-edge approach that can swiftly respond to emergencies and disruptions. The primary goal is to adapt scheduling strategies based on real-time data, considering collaborative utilisation of resources and human factors. By addressing unforeseen challenges such as sudden job insertions, machine breakdowns, and operator unavailability, the proposed approach seeks to minimise tardiness in production tasks. This innovation will enhance decision-making in job sequencing, machine selection, and worker assignment, ultimately leading to improved operational efficiency, enhanced worker satisfaction, and reduced costs within dynamic manufacturing environments.
https://www.cranfield.ac.uk/Research/PhD
Challenges in Dynamic Manufacturing:
The manufacturing landscape is not without its challenges. Unforeseen events like sudden job insertions or machinery breakdowns can disrupt production plans, highlighting the need for dynamic multi-objective rescheduling methods. Traditional methods, relying on genetic algorithms and dispatching rules, provide near-optimal results but lack efficiency and long-term efficacy. Moreover, challenges arise in scheduling collaborative resources while optimizing production time, resource utilisation, and meeting desired production goals. The influence of human factors, such as worker fatigue and skill levels, is often overlooked in existing approaches, limiting their adaptability in coping with uncertain dynamic disruptions within complex manufacturing environments.
Innovative Solution:
The core focus of this project is to address the limitations of existing dynamic scheduling approaches in complex manufacturing systems. The research will develop an innovative solution that integrates multi-agent systems and deep reinforcement learning techniques. Multi-agent systems, consisting of autonomous units making collaborative decisions, allow for quick responses to disruptions. Deep reinforcement learning, leveraging advanced algorithms and neural networks, learns optimal decision-making strategies from trial and error. Combining these approaches, the project aims to create a self-organising system that can dynamically adapt scheduling strategies based on real-time data. This approach will consider collaborative resource utilisation and human factors to minimise makespan, optimise resource utilisation, and provide real-time responsiveness to unforeseen disturbances.
Anticipated Outcomes:
The anticipated outcomes of this research hold substantial promise for the field of dynamic manufacturing scheduling. The integration of deep reinforcement learning and multi-agent systems is expected to lead to the development of an adaptive self-organising solution. This solution will optimise scheduling strategies based on real-time data and consider collaborative resource utilisation and human factors to mitigate tardiness in production tasks. The expected results include the development of a self-organising multi-agent system for resource scheduling, a deep reinforcement learning model for job sequencing and machine selection, and an attention-based network for worker assignment decisions.
https://www.cranfield.ac.uk/Research/PhD
Advantages of the PhD Programme:
The self-funded PhD programme at Cranfield University offers numerous advantages for aspiring researchers. It is centered on applied research that not only advances academic knowledge but also contributes to solving real-world challenges in smart manufacturing. The programme provides diverse training experiences, both internally and externally, enriching the researcher’s skill set and expanding their knowledge base. Cranfield University, renowned for academic excellence, provides a platform for unlocking promising career pathways. Interactions with experts from academia and industry foster extensive networking and offer exposure to cutting-edge insights. This collaborative environment nurtures personal growth and equips researchers with valuable connections within their field.
Entry Requirements and Funding:
Applicants with a First or upper Second Class degree equivalent qualification in an engineering background or a related quantitative discipline are invited to apply. The PhD opportunity is self-funded and open to UK, EU, and international applicants.
How to Apply:
To apply for this PhD opportunity, interested candidates can complete the application form on the Cranfield University website. For further information, applicants can contact Christina Latsou via email at christina.latsou@cranfield.ac.uk.
Embark on a journey of innovation and discovery by contributing to the advancement of smart manufacturing through Optimising Dynamic Scheduling with Reinforcement Learning. Cranfield University’s PhD programme offers a unique opportunity to shape the future of manufacturing operations and make a lasting impact on the industry.
https://scholarhubedu.com/financial-aid-a-guide-to-funding-your-education-in-uk-universities
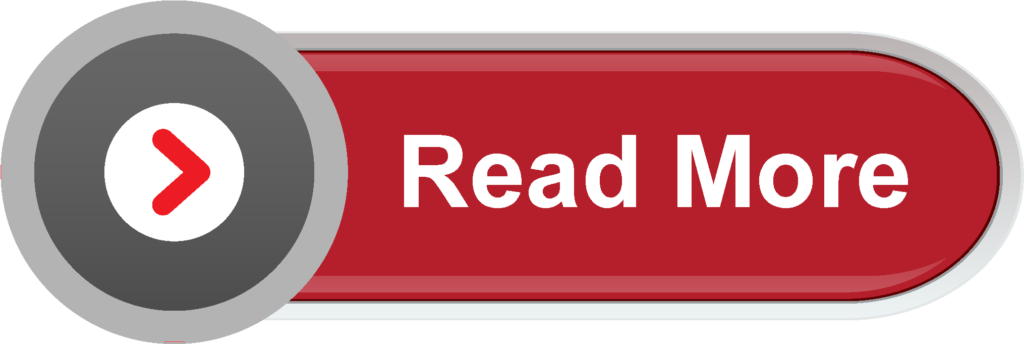